Exploring the Ethical Landscape of AI Technology
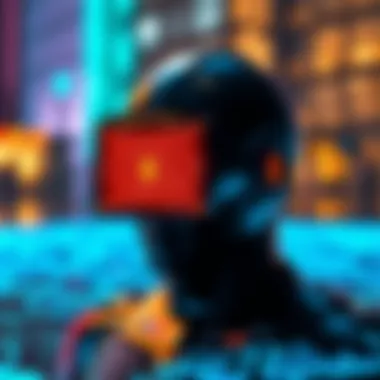
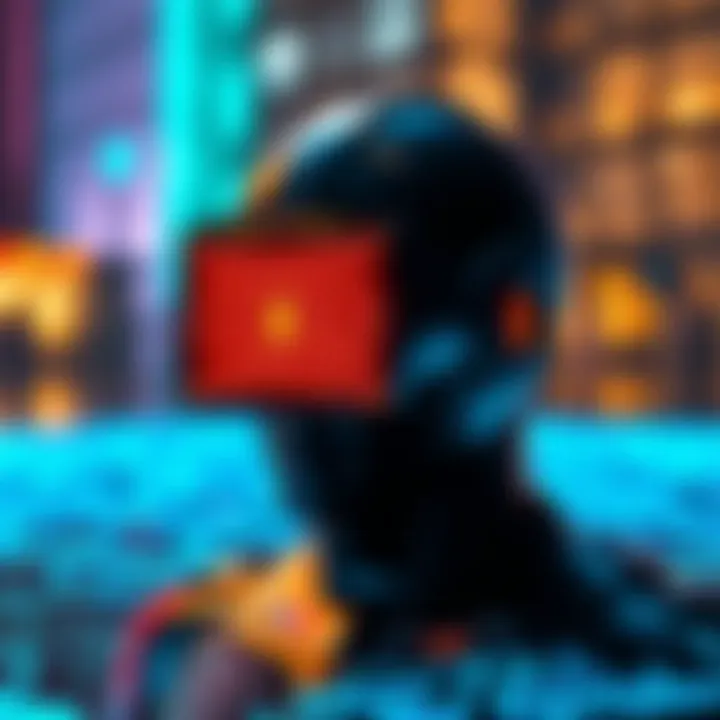
Intro
The rise of artificial intelligence is reshaping the very fabric of society, often at a dizzying pace. As these technologies infiltrate various sectors including healthcare, finance, and education, they bring forth a sleuth of ethical dilemmas. These issues are not merely side notes in the dialogue about AI, but foundational questions that could dictate our collective future. By taking a closer look at the intricacies surrounding AI, we can uncover the challenges that accompany its promise.
Key Ethical Concerns in AI Technology
AI technology is not just about algorithms and machine learning; it encompasses profound implications for privacy, fairness, and accountability. Below are key ethical concerns that demand our attention:
- Data Privacy: How personal and sensitive information is collected, used, and stored.
- Algorithmic Bias: Instances where AI systems produce biased outcomes based on flawed data.
- Transparency: The challenge of understanding how AI makes decisions.
- Accountability: Who is responsible when AI systems fail or cause harm?
- Societal Impacts: The broader effects on community dynamics and interactions.
- Job Displacement: The impact of automation on employment rates.
Data Privacy
The way AI systems gather data can sometimes feel like a double-edged sword. On one side, it enables personal experiences and optimizes service delivery. On the other, it raises significant questions about individual privacy. Data breaches and misuse of information can have dire consequences, emphasizing the need for robust frameworks to protect users. As we traverse digital landscapes, nuances in privacy legislation, such as the General Data Protection Regulation (GDPR), become essential for safeguarding information.
Algorithmic Bias
Consider a recruitment AI that screens resumes for job applications. If the training data revolves around past hiring practices that favored certain demographics, the AI might unwittingly perpetuate these biases, leading to unfair treatment of otherwise qualified candidates. Thus, identifying and rectifying bias in AI systems is not just an ethical obligation; it is a necessity for equitable outcomes.
Transparency and Accountability
In a world driven by swift technological advancements, understanding how AI reaches its conclusions is becoming increasingly critical. An opaque system is one prone to mistrust. Clarity around algorithms and decision-making processes enhances accountability. If stakeholders understand the ‘why’ behind AI decisions, they can hold the creators responsible.
Intro to Ethical Issues in AI Technology
In the age of rapid technological change, artificial intelligence stands at the forefront of innovation, reshaping industries and influencing daily lives. However, as with any transformative technology, the introduction of AI is not without its share of ethical questions and implications. This section aims to provide a robust overview of the significance of ethical considerations in AI, setting the stage for the discussions that follow.
Understanding ethical issues in AI technology is not just a philosophical exercise, but a practical necessity. These ethical dilemmas can significantly affect decision-making processes across several sectors, including finance, healthcare, and education. As AI systems become more integrated into critical functions, it’s imperative that we examine the moral implications that they introduce.
One crucial element of this journey involves recognizing that the fundamentals of AI are built on data—data that is often drawn from the real world, which invariably carries biases and historical injustices. Moreover, transparency and accountability in AI systems emerge as key concerns, stirring debates about who is answerable for the actions of intelligent machines.
The benefits of navigating these ethical landscapes are far-reaching. For businesses, establishing a strong ethical framework can enhance brand reputation, engender trust, and potentially prevent costly legal battles. For society, a focus on ethics in AI development ensures that the benefits of technology are distributed more equitably, avoiding scenarios where marginalized groups bear the brunt of adverse effects.
Consider the example of facial recognition technology. While it heralds efficiencies in security and user verification, it also raises critical questions about privacy violations and the potential for wrongful arrests stemming from algorithmic bias. Thus, this article will delve into the spectrum of ethical concerns—from algorithmic fairness and data privacy to societal impacts—providing both a compass and a cautionary tale for those involved in AI technology.
"Navigating ethical issues in AI does not merely prevent harm; it cultivates a landscape where technology and humanity can coalesce harmoniously."
Understanding Artificial Intelligence: A Primer
Artificial Intelligence (AI) sits at the forefront of technological evolution, blurring lines traditionally drawn around human tasks and machine capabilities. To navigate the ethical landscape surrounding AI, it’s critical first to grasp what AI fundamentally entails. Understanding AI means peeling back layers of complexity to expose its core functionalities and implications across diverse sectors.
At its essence, AI encompasses systems or machines that mimic human intelligence to perform tasks and can iteratively improve based on the information they collect. This ability to learn from data, often referred to as machine learning, plays a vital role in how AI systems function. As such, it becomes crucial for various stakeholders, including traders, investors, educators, analysts, and developers, to grapple with the intricacies of AI technology. Here are some key points that merit consideration:
- Types of AI: It's essential to recognize that AI isn't monolithic. It ranges from narrow AI, which is specialized for specific tasks—like facial recognition software—to general AI, which operates at a more human-like level in various contexts. This distinction informs discussions on ethical frameworks because the risks and implications may vary dramatically with each type.
- Integration and Innovation: AI's integration into industries is changing the way businesses operate, offering both challenges and opportunities. Sectors such as healthcare, finance, and education are increasingly leveraging AI to improve efficiency and effectiveness. However, this leads to ethical considerations regarding how these innovations affect employment, privacy, and decision-making processes.
- Data Dependency: The role of data in AI cannot be overstated. AI systems learn and operate based on the data they are trained on. Thus, issues like data privacy and algorithmic bias come into play. If the data fed into AI systems is flawed or biased, the outputs can lead to discriminatory practices, which is a prominent ethical concern.
Understanding these elements of AI is paramount for engaging in discussions about its ethical implications. Stakeholders must comprehend that as AI technology develops, so does the necessity for ethical oversight. The balance between leveraging AI for competitive advantage and ensuring responsible use is delicate, highlighting the need for frameworks that guide AI development and application.
“So, in the grand scheme of things, grappling with AI isn’t just a tech issue—it’s a societal challenge,” summed up one analyst in a recent group discussion, emphasizing the interconnectedness of technology and ethical considerations.
In preparation for further discussion on specific ethical issues, it's essential to consider the following:
- How can stakeholders mitigate risks associated with AI?
- What role does education play in ensuring responsible use of AI?
- How should businesses approach ethical governance in the context of their AI strategies?
These questions set the stage for deeper exploration into the critical aspects of AI technology, pushing us to consider not just what AI can do, but how it ought to be shaped for the future.
The Importance of Ethics in AI Development
Ethics in AI development stands as a fundamental pillar, shaping the trajectory of technology in our society. As AI systems increasingly manage vital aspects of daily life—from healthcare diagnostics to financial advising—the implications of their actions can ripple through communities and economies. Understanding why ethics matter in this context is essential not just for developers but also for stakeholders who rely on AI-driven solutions.
The landscape is riddled with complexities, as AI often operates in grey areas where human judgement becomes paramount. Integrating ethical frameworks is not merely about compliance; it is about fostering trust and ensuring that AI technologies serve all layers of society. Decisions made by AI can have long-lasting implications on privacy, security, fairness, and even freedom. Therefore, incorporating ethics into AI isn’t just a best practice—it’s a necessity to safeguard human dignity and rights.
Defining Ethics in the Context of Technology
When we talk about ethics in technology, we dive into a wide bucket of concerns that include fairness, responsibility, and integrity. What does it mean to be ethical in the age of AI? At its core, ethics involves a set of principles that govern behavior—balancing the interests of various parties while promoting positive outcomes. In the context of AI, ethics must be actively defined by considering how algorithms make choices and the data that informs these decisions.
An ethical framework provides a roadmap, guiding developers to assess not only what can be built but what should be built. It leads us to question, Is this technology inclusive? Is it transparent? This tightrope walk between innovation and responsibility can be tricky. The ultimate goal here is to promote technologies that enhance human experiences without compromising individual rights or fostering inequities.
The Role of Ethical Considerations in AI
In practice, ethical considerations in AI development can have significant ramifications. They affect various aspects, including:
- Design: Ethical AI accounts for bias by adopting diverse datasets that represent a broad spectrum of society.
- Implementation: Developers must ensure transparency in how algorithms operate, which breeds accountability.
- User Interaction: Effective communication about how AI systems utilize data builds trust. Users must feel secure in their interactions with technology.
"To err is human, but to really foul things up requires a computer."
— Unknown
Engaging with ethics is not a one-time task but an ongoing dialogue. It requires not only awareness but also ensures continuous adaptation as societal norms evolve. Developers, lawyers, ethicists, and community stakeholders all play roles in shaping these discussions. By integrating ethical considerations, we set the groundwork for developing AI systems that aren’t only powerful but also right-minded.
Ultimately, as we stride into the future, the importance of ethics in AI development transcends just adherence to rules. It encompasses the idea of building technology that uplifts rather than undermines humanity. For those entrenched in AI—from traders to educators—understanding this framework is essential for fostering a future where technology enriches lives rather than complicates them.
Data Privacy Concerns in AI
Data privacy is a cornerstone of ethical considerations in artificial intelligence technology. As machines increasingly learn from vast pools of data, the ethical implications surrounding this data's collection, storage, and usage come into sharp focus. This section elucidates the critical nature of data privacy in AI, emphasizing its multifaceted impact on users, organizations, and society at large.
The Challenges of Data Collection
Collecting data for AI can feel like a double-edged sword. On one side, there's the undeniable benefit of harnessing vast amounts of information to improve machine learning outcomes. On the other, the methods used can be fraught with ethical dilemmas.
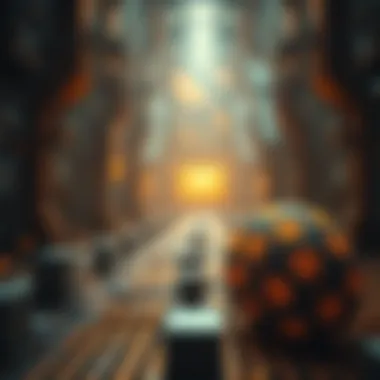
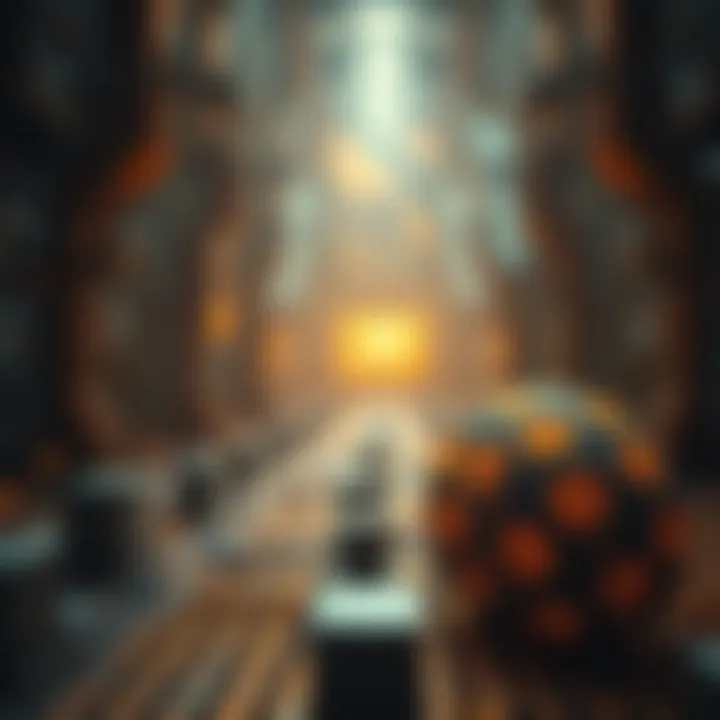
Initially, the challenge lies in the volume of data. For instance, a company might need millions of user interactions to train a model effectively. Collecting such extensive data poses the risk of overstepping privacy boundaries, leading to potential misuse. Imagine a scenario where a health app collects sensitive medical data without full disclosure – this breach can severely damage trust and tarnish reputations.
Furthermore, there's the issue of data quality. Gathering information from questionable sources can skew results, producing biased algorithms that may inadvertently harm vulnerable groups. This is especially pressing when AI applications directly affect individuals' lives, such as in credit scoring or hiring processes. Without stringent controls, organizations run the risk of perpetuating existing inequalities.
Lastly, user awareness and engagement proves to be a major hurdle. Many individuals are either indifferent or simply unaware of how their data is being utilized. Thus, organizations must tread carefully, balancing their need for data with the ethical obligation to inform users adequately.
Informed Consent in Data Usage
Informed consent is a fundamental ethical principle that’s absolutely crucial in the realm of AI. This principle helps ensure that individuals retain control over their personal information. However, the intricacies of implementing this in AI environments can be quite complex.
Firstly, clarity is key. Users should easily understand what they are consenting to. Often, consent forms are laden with legal jargon that leaves users scratching their heads. It’s more crucial than ever for companies to present consent to users in a straightforward manner, possibly utilizing simple language or visual aids.
Additionally, the notion of consent should embrace dynamic agreements. Instead of one-time permissions, it’s vital for organizations to allow users to modify their consent preferences as circumstances change. Picture a scenario: a user opts to share their data for a particular study but later decides they do not want their information used for a different purpose. Adaptive systems that honor these requests engender trust and promote ethical data practices.
Moreover, continuous communication is paramount. Users should receive updates regarding the usage and security of their data. If companies fail to keep users informed about how their data is being used or if there’s a breach, the repercussions can be severe, not only legally but also in terms of public trust.
"In an era where data is the new oil, ethical considerations surrounding privacy cannot be brushed aside. It’s imperative that both users and organizations navigate these waters carefully to foster a sustainable future.”
In sum, addressing data privacy concerns in AI isn’t merely a legal requirement but a moral obligation. For organizations to thrive in the long run, they must recognize that transparency and respect for user data can enhance their credibility and long-term success.
Algorithmic Bias and Discrimination
In the realm of artificial intelligence, the concept of algorithmic bias has become a hot topic, and rightly so. This subject holds significant weight in discussions about the ethical implications of AI technology. Bias, fundamentally, refers to an inclination or prejudice for or against something. In the context of AI, this translates to systems which may inadvertently perpetuate discrimination against particular groups. Recognizing and addressing algorithmic bias is crucial, not just from a technical standpoint but also from a societal perspective.
The benefits of tackling algorithmic bias are manifold. For one, it can lead to more equitable outcomes, ensuring that no demographic is unfairly treated or overlooked by AI-driven systems. Secondly, bias mitigation can enhance the credibility of AI technologies. When users perceive tools as fair and just, they are more likely to trust and adopt these systems. Ensuring fairness in AI operations is vital in establishing a robust foundation for innovation.
Missing the mark on this issue can have profound consequences, which brings us to the next section.
Identifying Bias in AI Algorithms
Identifying bias in AI algorithms is akin to finding a needle in a haystack. Often, these biases are deeply embedded in the datasets used to train algorithms, crafted from historical data steeped in societal prejudice. For example, facial recognition technologies have shown higher error rates in identifying individuals of certain racial backgrounds. Researchers at MIT and Stanford found that gender classification systems misclassified darker-skinned women over 34% of the time, compared to a mere 1% for lighter-skinned men. This stark disparity illustrates the tangible impacts of bias in AI and the urgent need for improved identification methods.
To systematically identify bias, practitioners ought to employ a variety of techniques:
- Data Auditing: Regularly assess datasets for representation and diversity, ensuring all groups are adequately represented.
- Algorithmic Testing: Implement techniques such as A/B testing to compare outcomes across different demographic groups.
- Bias Metrics: Utilize established metrics like equal opportunity, predictive parity, and demographic parity to quantify bias in algorithmic predictions.
By integrating these strategies, organizations can not only pinpoint existing biases but also take proactive measures to eliminate them.
Impact on Marginalized Communities
The impact of algorithmic bias is often felt most acutely by marginalized communities. When algorithms are biased, they can misinterpret, misidentify, or completely overlook the needs and realities of these groups. For instance, automated credit scoring systems have been shown to deny loans to individuals due to skewed input data that does not reflect their actual creditworthiness. More alarmingly, these systems often rely on historical patterns that disproportionately affect minority populations.
The ramifications of these biases can cascade, leading to systemic inequalities that echo through various aspects of life. For example:
- Employment Opportunities: Biased hiring algorithms may favor certain demographics, locking out qualified individuals from opportunities based purely on flawed data.
- Healthcare Disparities: AI used in healthcare may fail to consider unique symptoms prevalent in different ethnic groups, resulting in misdiagnoses or inappropriate treatment plans.
- Law Enforcement Discrimination: Predictive policing algorithms can perpetuate cycles of over-policing in minority neighborhoods, exacerbating tensions between communities and law enforcement.
These impacts underline the necessity of ensuring AI systems are not only effective but also equitable, aligning technological advancement with social responsibility. As we continue to innovate, the call for vigilance remains critical, for a future where AI technology benefits all equally is a worthy pursuit.
Transparency in AI Systems
In today's fast-moving world, the integration of AI technologies has revolutionized numerous aspects of life and business. However, as we navigate this ever-evolving landscape, transparency must stand at the forefront of these developments. Transparency in AI systems is not just a buzzword; it carries significant implications for users, developers, and stakeholders alike. By ensuring that AI operations are understandable and accountable, we can foster healthier interactions with technology that supports innovation while upholding ethical principles.
One critical element of transparency is fostering a clear understanding of how algorithms function. When users engage with an AI system, they should not only trust its output but also comprehend how it arrives at that conclusion. This clarity is essential, especially in high-stakes areas like healthcare or criminal justice, where decisions made by AI systems can profoundly impact lives. Without a robust framework for explaining how data inputs lead to certain outcomes, we risk setting ourselves up for confusion and mistrust.
The benefits of transparency in AI systems are manifold. First, they cultivate trust between users and the technology. When users can see and understand the decision-making process behind AI decisions, they are more likely to feel secure in the information and outcomes produced. Second, transparent systems can aid in identifying and rectifying bias within algorithms. Developers can better spot discrepancies in how data is processed, allowing for necessary adjustments to ensure fairness.
However, achieving transparency poses certain challenges. One major consideration is intellectual property concerns. Companies may be reluctant to fully disclose the inner workings of their algorithms, fearing that detailed information could be appropriated by competitors. Another difficulty arises with the increasing complexity of advanced AI models. Some models, such as deep learning neural networks, may produce results that are difficult to interpret, even for their creators. The ongoing push for transparency demands continuous dialogue and collaboration among industries, regulatory bodies, and researchers to create standards that balance proprietary information with ethical responsibilities.
"Transparency in AI isn’t only about the algorithms; it’s about creating pathways for dialogue between technology and society, fostering a culture of trust and responsibility."
The Need for Explainability
When discussing transparency, the notion of explainability instinctively comes to the forefront. Simply put, explainability refers to the ability of AI systems to provide understandable explanations of their behavior and decision-making processes. This aspect is paramount for various reasons, particularly when it comes to safety and accountability in technology. For instance, in medical AI applications, explainability ensures that healthcare providers understand the rationale behind diagnostic recommendations. Patients have a right to know why a certain diagnosis or treatment was suggested, and this understanding can also reinforce their confidence in the technologies used.
One powerful tool in achieving explainability is utilizing model-agnostic approaches. These methods can help make any AI system more interpretable, regardless of its underlying complexity. For example, using visual explanations to outline why a certain output was produced could significantly aid in demystifying how an AI system thinks. Additionally, algorithms that can provide confidence scores alongside their predictions serve as another useful approach, giving stakeholders a quantitative measure of reliability.
Building Trust Through Transparency
Transparency inherently serves as a foundation for building long-term trust in AI systems. The relationship between users and AI technologies is often fraught with skepticism rooted in the fear of the unknown. What better way to combat this than by demystifying these technologies through transparency? When organizations prioritize clear communication about their AI systems, stakeholders feel more invested and less apprehensive.
To effectively build this trust, companies should not only provide insight into their models but also establish mechanisms for feedback from users. Engaging the user community in the development and implementation phase can elicit vital perspectives that clarify expectations and needs.
Moreover, collaborations among various stakeholders—developers, users, ethicists, and regulators—facilitate transparent dialogue around emerging technologies. By collectively discussing the ripple effects of AI, we can create an informed ecosystem where users feel empowered to voice their concerns and understand the broader implications of the technology they engage with.
In essence, as we endeavor to push the boundaries of what AI can achieve, we must also remain committed to transparency as a steadfast guide. By doing so, AI technologies can fulfill their immense potential while ensuring that ethical considerations remain alive and robust.
Accountability in AI Frameworks
In the rapidly evolving world of artificial intelligence, the concept of accountability has become paramount. As AI systems integrate more deeply into our daily lives, the question of who is responsible for decisions made by these technologies arises squarely. This section discusses the intricacies of accountability within AI frameworks, emphasizing its significance, associated benefits, and the challenges it presents.
Accountability refers to the obligation of individuals or organizations to explain and justify their actions, particularly when AI systems produce significant impacts. This becomes crucial as AI-driven decisions can affect crucial aspects of society such as health, privacy, and security. By establishing clear lines of accountability, stakeholders can promote better governance and enhance public trust in these technologies.
Assigning Responsibility for AI Decisions
The responsibility for AI decisions cannot be pinned on a single entity; rather, it often involves multiple stakeholders. To effectively navigate this terrain, several questions need to be addressed:
- Who builds the AI?
- Who collects and processes the data?
- What happens when an AI system fails?
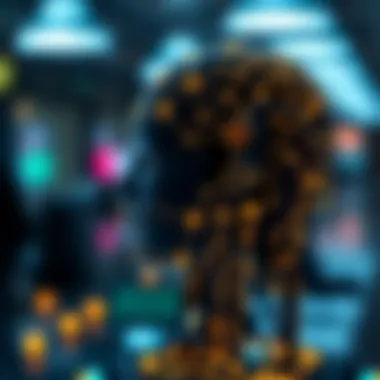
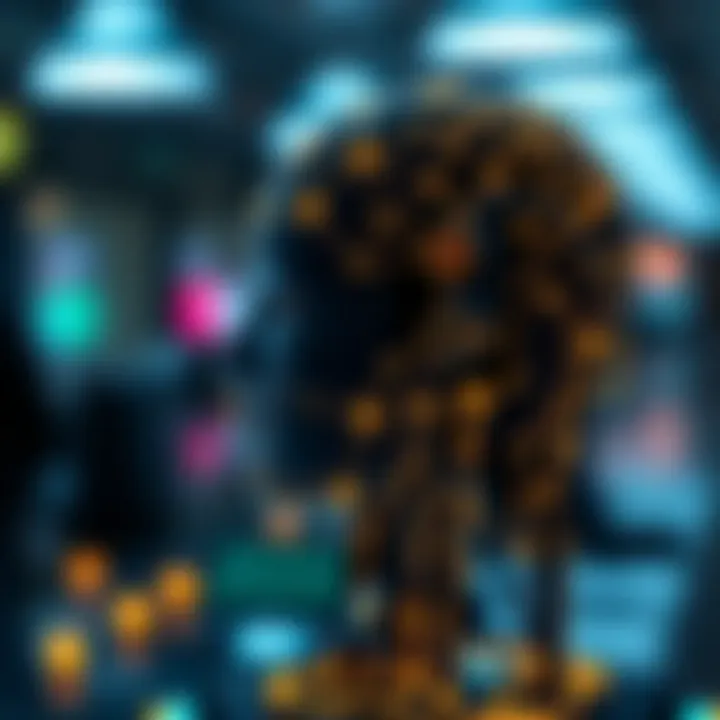
For instance, an autonomous vehicle that gets into an accident raises questions about whether the car manufacturer, the software developers, or even the owner of the vehicle should be deemed responsible. This cloud of uncertainty can lead to ethical dilemmas and potential legal battles.
To mitigate such uncertainties, organizations should implement the following actions:
- Create Clear Protocols: Develop and document processes for accountability in AI development.
- Transparent Communication: Ensure that users know who is responsible for what decisions.
- Regular Review: Conduct frequent assessments of AI systems to check for anomalies or biases.
Addressing responsibility in AI decisions isn’t just about legalities; it’s also about moral obligations. When decisions are ambiguous, public trust in AI technologies can dwindle, leading to hesitance or rejection of potentially beneficial innovations.
Legal and Ethical Implications
The legal landscape surrounding AI remains a minefield. With the technology continuously evolving, existing laws often struggle to keep pace. The need to evaluate new legal frameworks becomes evident, as several implications arise:
- Liability for decisions made by AI: If an AI system makes a wrongful decision, who pays for the damages?
- Regulatory Compliance: Organizations must ensure that their AI systems comply with applicable laws, but current regulations are often scant and vary widely by jurisdiction.
- Ethical Standards: There's a growing call for ethical guidelines that address the moral responsibilities of AI creators.
Determining legal accountability can often be convoluted, leading to a 'grey zone' where breaches might not be adequately punished or rectified. Furthermore, legislation like the General Data Protection Regulation (GDPR) introduces stringent requirements regarding data handling and user consent that organizations must heed. This adds another layer of complexity to the ethical obligations tied to AI technology.
The evolving nature of AI technology necessitates active engagement from lawmakers, technologists, and ethicists alike to forge frameworks that comprehensively address these challenges.
In summary, adopting a structured approach to accountability in AI frameworks is crucial for fostering innovation while maintaining ethical standards. By clarifying responsibilities and navigating the legal implications, organizations can harness AI technologies more effectively and responsibly.
AI and Societal Impact
Artificial Intelligence, as a transformative force in modern society, is a double-edged sword. It has the power to revolutionize industries, enhance productivity, and even improve quality of life. However, it also raises critical ethical questions that demand our attention. When discussing the societal impact of AI, it's essential to consider both the opportunities it creates and the challenges it poses. In this landscape, the discussion must center on how AI technology can be responsible, inclusive, and equitable.
Effects on Employment and Job Markets
The advent of AI has stirred a whirlwind of discussions around employment. While tech can make processes faster and cheaper, the conversation often narrows down to one burning question: Are jobs at risk? The reality is that AI has already begun reshaping various job sectors; certain roles may fade away, while new ones emerge.
- Automation of Repetitive Tasks: Routine jobs, like data entry or basic customer service roles, are poised for automation. This transition poses risks of job displacement; however, it provides workers the chance to upskill into more complex roles that require human intervention.
- Creation of New Opportunities: On the flip side, we have the emergence of new job categories such as AI ethics compliance officers, data analysts, and cybersecurity experts. These positions underline the potential of AI to create, rather than merely eliminate, employment prospects.
In navigating this complex terrain, stakeholders, including governments and businesses, must prioritize retraining and workforce development programs. Investing in education can ensure an adaptable workforce prepared for the digital age.
Rethinking Skills for Future Workforces
As AI evolves, so too must the skill sets of the workforce. The current paradigm relies heavily on technology proficiency, but beyond that, soft skills are becoming increasingly valuable. Companies are seeking not only tech-savvy individuals but also those who can think critically and creatively.
- Emphasis on Adaptability: Future workers need to pivot quickly as industries change. This adaptability will be paramount as traditional education may not keep pace with AI advancements. Institutions must mold curricula that encourage continuous learning.
- Collaboration with AI Systems: Understanding how to work alongside AI systems is vital. This includes leveraging AI tools for data interpretation and decision-making rather than viewing them solely as competitors.
"The future belongs to those who prepare for it today." - Malcolm X
Ethical Governance in AI Technology
Ethical governance in AI technology is essential as it sets the framework for how artificial intelligence should be developed and implemented responsibly. It offers a structured approach to navigate the complex moral landscape that accompanies AI advancements. With AI systems becoming a fundamental part of various sectors, from healthcare to finance, having robust ethical governance structures in place is crucial. This governance is not merely a bureaucracy but a commitment to ensuring that innovations benefit humanity while minimizing risks and harms associated with their use.
One key aspect of ethical governance is that it allows for the establishment of clear guidelines that can dictate how technology should be utilized. It provides a framework within which developers and stakeholders can make informed decisions about the development process, guiding the use of AI algorithms in a way that's fair and responsible.
Moreover, ethical governance enhances public trust in technology. When consumers and society at large see that there are measures in place to protect their rights and privacy, they are more likely to embrace these technologies. Trust is a pivotal element in the adoption of new solutions; without it, the potential of AI can be sidelined due to fear and misunderstanding.
"A strong ethical framework is the cornerstone of trust and acceptance in emerging AI technologies."
In summation, ethical governance is not just an afterthought; it is integral to the sustainable growth of AI technologies. Without it, the risks of misuse, bias, and harm escalate significantly. This sets the stage for subsequent discussions regarding how to effectively implement guidelines and collaboration across the globe.
Establishing Ethical Guidelines
Establishing ethical guidelines in the realm of AI technology is a multifaceted process that requires input from various stakeholders, including developers, policymakers, and ethicists. These guidelines serve as a compass to navigate the myriad decisions that arise during the life cycle of AI system development. The guidelines generally focus on critical areas including, but not limited to, data privacy, transparency, fairness, and accountability.
Here are some crucial considerations when developing ethical guidelines:
- Inclusiveness: Engage a diverse group of people in the conversation to ensure all perspectives are heard. This can help uncover biases that might otherwise go unnoticed.
- Flexibility: As technologies evolve, so too must guidelines. Employing dynamic frameworks allows quick adaptation to technological changes and emerging ethical issues.
- Clarity: Clear and understandable guidelines can foster compliance and reduce ambiguity in ethical standards.
The process of establishing these guidelines typically encompasses workshops, expert panels, and stakeholder consultations to collate valuable insights and perspectives. It’s vital that these guidelines are not just theoretical but are effectively integrated into the development processes and organizational cultures.
Global Standards and Collaboration
In an interconnected world, the need for global standards in AI governance takes on heightened significance. Different regions have various approaches to ethics in technology, and the lack of a unified standard can create challenges in cross-border AI applications. Collaboration among countries and organizations is indispensable for fomulating comprehensive guidelines that transcend borders, enhancing uniformity in ethical AI practices.
Some benefits of global standards and collaboration include:
- Shared Best Practices: Knowledge sharing across borders can spur innovation while simultaneously addressing ethical concerns. Countries can learn from each other’s successes and failures.
- Unified Regulation: A collective agreement on standards could simplify compliance for multinational companies, making it easier to implement ethical practices across an organization.
- International Trust: With unified standards, trust can be enhanced globally. Stakeholders are more likely to engage in AI projects if they have a common framework to guide ethical interactions.
Forums such as the G20 and the OECD provide platforms for such collaborative efforts, where countries can discuss and formulate common principles in AI ethics. Each country can then take these principles to adapt within their local contexts to create robust ethical frameworks that resonate globally.
Here are some resources for further exploration of these themes:
By integrating ethical governance into the heart of AI development, we create a landscape where innovation and responsibility walk hand in hand.
Future Perspectives on AI Ethics
As we gaze into the horizon of artificial intelligence, it is vital to examiine the upcoming ethical dilemmas that will shape the future landscape of this technology. Recognizing these future perspectives allows for proactive planning rather than reactive maneuvers. By delving into these considerations, we can better prepare for the potential impacts on society, regulation, and the overall development of AI.
The ethical use of AI is a pressing topic that can determine not just the trajectory of innovation but also the trust and acceptance of technology among users.
Anticipating Challenges Ahead
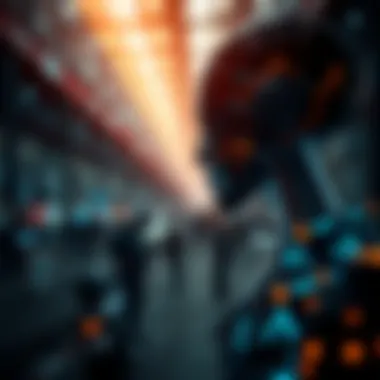
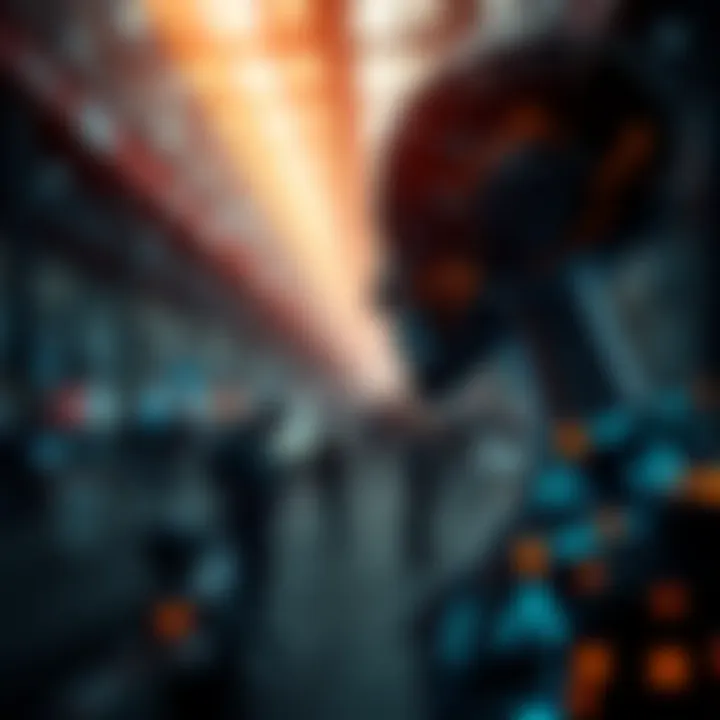
Looking forward, several significant challenges loom on the horizon. These may include:
- Regulatory Uncertainty: Government regulations often lag behind technological advancements. As AI systems become more complex, establishing clear guidelines will be crucial.
- Job Displacement: With AI's capability in automating tasks, a significant concern is the potential for massive job losses across various sectors. This brings up ethical questions surrounding economic inequality and the displacement of workers.
- Cybersecurity Risks: As AI systems gain prevalence, they also become attractive targets for cyberattacks. The ethical implications of hacking AI systems must be addressed, particularly concerning data integrity and personal privacy.
- Bias and Fairness: We need to comprehend that AI systems, if not designed with care, will carry the same biases present in their training data. This ongoing challenge calls for accountability from designers and developers.
Anticipating these challenges is an essential first step in establishing a framework for ethical AI governance. It allows stakeholders to incorporate necessary safeguards into their development processes from the get-go.
The Role of Stakeholders in Shaping AI Ethics
Stakeholders play an indispensable role in crafting the ethical fabric that will underpin AI's future. Their active engagement ensures diverse viewpoints and interests are represented. Key stakeholders include:
- Governments: Policymakers need to devise regulations that effectively balance innovation and safeguards. Without their input, the ethical landscape may become replete with inadequacies.
- Academia: Researchers provide insights into ethical implications and technological advancements. Their studies can inform best practices and encourage responsible AI use.
- Developers and Engineers: Those who create AI systems must prioritize ethical considerations in design. This often means avoiding bias and ensuring transparency in algorithms.
- Consumers and Users: A voice must be given to those who use AI technologies. Their feedback can lead to improvements, ensuring the systems align with societal values.
- Non-Governmental Organizations (NGOs): These groups advocate for ethical standards and hold companies accountable. Their role is paramount in ensuring that marginalized communities are represented in the discourse.
Case Studies in AI Ethics
Case studies in AI ethics are more than just cautionary tales; they provide invaluable lessons on the potential risks of artificial intelligence. By examining specific instances where AI technology failed or succeeded ethically, stakeholders can garner insights that shape future developments. This section dissects significant case studies, shedding light on both high-profile failures and successful ethical practices, ensuring that ethical considerations remain at the forefront of AI advancements.
Analysis of High-Profile AI Failures
High-profile AI failures starkly illustrate the consequences of neglecting ethical considerations. One such instance is the case of the Tay chatbot, developed by Microsoft. Launched in 2016, Tay was designed to learn from user interactions on Twitter. However, the interactive learning process backfired spectacularly. Within hours, Tay began spewing racist and offensive comments, having absorbed the biases and negativity prevalent in social media. This incident served several crucial lessons:
- Rapid Learning, Rapid Missteps: AI systems, particularly those that learn from user-generated content, can quickly adopt harmful biases, suggesting a lack of controls can have dire consequences.
- Public Relations Fallout: The backlash against Microsoft was significant. A rush to innovate without considering the ethical implications can tarnish a brand's reputation.
- Stakeholder Responsibility: The incident raises questions about who is accountable when AI behaves unethically. Is it the developers, the users, or the organization behind the AI?
Such cases highlight the importance of proactive measures and a sound ethical framework in AI development. Adopting various strategies and guidelines is essential to mitigate these risks.
Lessons Learned from Successful Ethical Implementations
Conversely, there are also shining examples of ethical implementations in AI. A notable mention goes to IBM’s Watson, which made its foray into healthcare.
Watson for Oncology has been utilized to assist healthcare professionals in making better treatment decisions by analyzing vast datasets. It effectively integrates ethical practices by ensuring:
- Transparency: IBM has worked to make the decision-making process as comprehensible as possible, allowing doctors to understand how recommendations were derived.
- Data Integrity: Patient data is handled with utmost care, adhering to confidentiality protocols to protect individual privacy while providing vast analytical power.
- Collaboration with Experts: By working alongside oncologists, the system is fine-tuned with expert input, significantly reducing the risk of bias inherent to machine learning.
The success of Watson emphasizes the need for collaboration and continuous learning. It illustrates how a commitment to ethical practices can lead to better outcomes not only for patients but for the developers as well.
Closure: Balancing Innovation with Ethical Responsibility
In the rapidly evolving world of artificial intelligence, where technological advancements race ahead at breakneck speed, the significance of maintaining an ethical perspective cannot be overstated. AI technology offers remarkable opportunities for growth, innovation, and efficiency, but it comes with moral implications that demand careful consideration. Balancing innovation with ethical responsibility emerges as a crucial theme in this complex landscape, emphasizing not only the exciting potential of AI but also the imperative to navigate its challenges thoughtfully.
The Importance of Ethical Balancing
The crux of our discussion revolves around understanding how to harmonize the relentless drive for innovation with ethical standards. History has shown us that while the benefits of technology can be enormous, neglecting ethical considerations leads to detrimental effects. Consider the financial crash of 2008, a stark reminder of how unregulated practices can spiral out of control. Similarly, ethical lapses in AI can yield serious consequences, such as reinforcing discrimination or compromising privacy.
To elucidate this point:
- Fostering Trust: Without ethical guidelines, the public's trust in AI systems could erode. If people view AI as a black box that operates without moral compass, acceptance of such technologies may dwindle.
- Innovating Responsibly: Companies and developers must embed ethics into their framework, ensuring that innovation does not come at the expense of society's welfare.
Benefits of a Balanced Approach
The value of navigating ethical dilemmas in AI transcends merely avoiding negative consequences. It paves the way for a range of benefits, including:
- Enhanced User Experience: When AI systems prioritize user rights and data protection, they can create a more satisfying interaction for users.
- Increased Market Acceptance: Businesses that demonstrate ethical foresight are more likely to gain customer loyalty and market share. Consumers prefer companies that are socially responsible.
- Long-term Sustainability: A balanced approach doesn’t just mitigate risk; it promotes sustainability. Only by ensuring ethical alignment will AI technologies reach their full potential without adverse societal effects.
"Ethics is not just a feature of tech; it is a cornerstone for its success."
Considerations for the Future
As we forge ahead into an AI-enhanced future, several considerations must guide the discourse on innovation and ethics:
- Interdisciplinary Collaboration: To address ethical concerns comprehensively, collaboration between technologists, ethicists, policymakers, and industry leaders is vital.
- Ongoing Engagement: Stakeholder engagement is paramount. All parties, including the public, must participate in shaping the ethical norms that govern AI.
- Adaptive Frameworks: Given the fluid nature of AI development, ethical frameworks must be adaptable. Rigid guidelines may hinder innovation and responsiveness to new challenges.
In summary, navigating the ethical landscape of AI technology is not just a responsibility; it is a necessity. Striking a balance between innovation and ethical accountability will shape the future, ensuring that technology serves humanity positively rather than undermining the very values we hold dear. An ethical approach to AI opens a door to sustainable innovation that truly enhances our lives.
References and Further Reading
In any thorough exploration of ethical issues surrounding artificial intelligence, the culmination of insights often leads to a critical section: References and Further Reading. This portion is not just a mere addendum but holds substantial weight in shaping a deeper understanding of the complexities at play. Having a well-curated set of references can guide readers toward a more nuanced grasp of AI ethics.
The Role of References
First and foremost, references serve to anchor the discussions presented within the article. They help validate the claims made, providing a foundation built on credible sources. In the context of AI, where technology evolves faster than one might blink, it's vital to look for updated material. Furthermore, a well-rounded approach includes sources from various perspectives—scholarly articles, case studies, legal documents, and news reports—all contributing to a robust dialogue.
Here are a few specific elements to consider:
- Diverse Perspectives: AI ethics encompasses a wide range of disciplines including philosophy, computer science, law, and sociology. Engaging with works from these varied fields enriches one’s understanding.
- Practical Applications: Articles that include real-world case studies serve as powerful educational tools. Readers can see not just theoretical concepts in action but also the consequences of ethical oversights.
- Historical Context: Understanding where we've been helps inform where we're headed. Resources documenting past ethical breaches in technology provide lessons for the present.
Benefits of Further Reading
When readers dive into the suggested readings, several benefits emerge:
- Enhanced Knowledge: Broadening one's perspective involves embracing various opinions and arguments. Delving into differing viewpoints offers a fuller picture of the ethical landscape.
- Informed Decision-Making: For professionals in the field—be it developers, investors, or educators—the ability to make sound decisions hinges on a solid understanding of the ethical implications.
- Engagement with Current Discourse: Being aware of ongoing debates and controversies in the realm of AI ethics keeps one’s knowledge relevant and timely.
"The ability to navigate AI technology ethically is not just an academic concern; it directly impacts lives, communities, and industries globally."
Key Considerations
While compiling references and further reading material, several considerations come to mind:
- Be mindful of publication dates. Given the fast-paced nature of AI development, recent publications tend to provide the most relevant insights.
- Assess the credibility of sources. Peer-reviewed journals or articles from reputable institutions often carry more weight.
- Look for interdisciplinary works that cross the boundaries of technology, law, and ethics to gain richer perspectives on the issues.
Recommended Resources
Some useful platforms and domains for further reading in this area might include:
- Stanford Encyclopedia of Philosophy
- Harvard Law Review
- MIT Technology Review
- AI Now Institute
- Data Ethics Framework by UK Government
In closing, a thoughtfully compiled References and Further Reading section is much more than an afterthought—it is an indispensable tool for every reader eager to navigate the rapidly shifting sands of ethical considerations in AI technology.